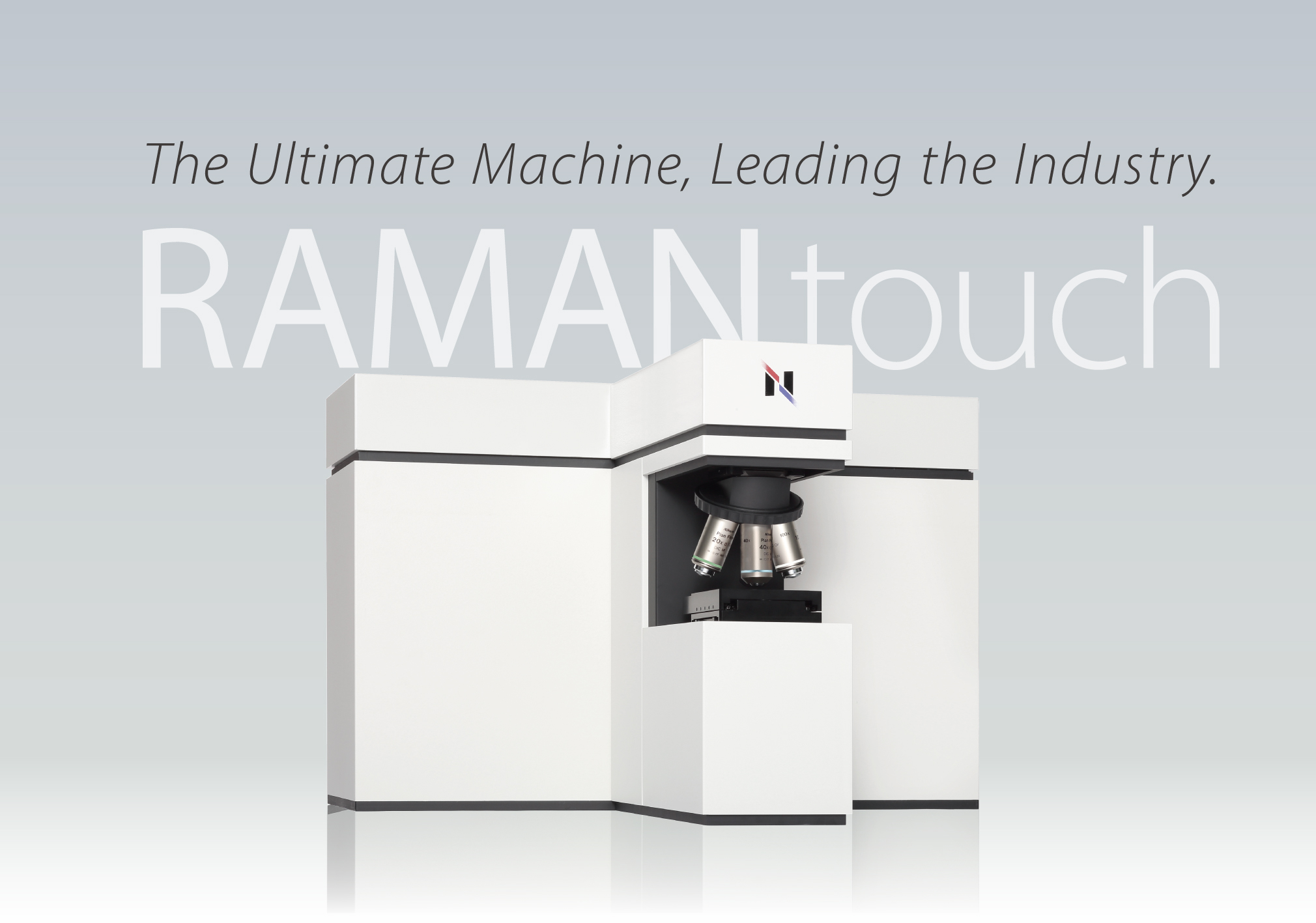
Nanophoton is the pioneer of confocal laser scanning microscopy and spectroscopic analysis. To celebrate, we relaunch our fastest and highest resolution confocal Raman microscope RAMANtouch/RAMANforce. With a newly designed spectrograph and the latest optical technologies, RAMANtouch/RAMANforce’s spatial resolution and sensitivity have been highly improved, making it the optimal solution for all applications.
Microscopy
The highest resolution at every objectives
RAMANtouch/RAMANforce has finally accomplished perfect resolution.
Resolution at 20x and lower objectives has been improved to be optimal using a newly designed spectrograph without aberration, while maintaining the highest resolution with the 100x objective.
The highest spatial resolution
RAMANtouch/RAMANforce is equipped with dedicated optics and a high-quality TEM00 laser for the highest spatial resolution. With a 532 nm excitation wavelength and 0.90 NA (Numerical Aperture) objective, RAMANtouch/RAMANforce guarantees 350 nm spatial resolution for all users. Raman imaging data of fluorescent beads of 200 nm in diameter is shown on the right. The intensity profile along the dotted line in the Raman image demonstrates a spatial resolution of up to 300 nm. The high spatial resolution also enhances the detection sensitivity of tiny objects that are smaller than the diameter of the laser spot. RAMANtouch/RAMANforce is thus able to detect a small particle under 100 nm diameter.
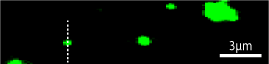
Sample | Fluorescent beads(φ200nm) |
Excitation wavelength | 532nm |
Objective lens | 100x, 0.90N.A. |
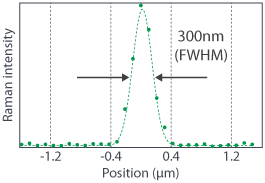
Furthermore, RAMANtouch/RAMANforce uses a newly designed spectrograph with aberration completely compensated for, which greatly improves spatial resolution of 20x and lower magnification objectives. Clear Raman imaging is always possible at each magnification.
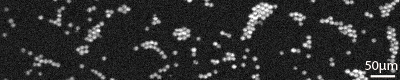
Sample | Fluorescent beads(φ10µm) |
Exciotation wavelength | 532nm |
Obj.lens | 10x, 0.30N.A. |
Depth resolution by dedicated confocal optics
Since RAMANtouch/RAMANforce is equipped with dedicated confocal optics, high spatial resolution is achieved even in the z-direction (depth into the sample). The data on the right show the cross-sectional Raman imaging of 200 nm diameter fluorescent beads. The intensity profile demonstrates a spatial resolution of 670 nm. Due to this confocal behavior, the focal point’s signal from the inner part of the transparent sample can be nondestructively measured. RAMANtouch/RAMANforce works as a perfect tool for cross-sectional Raman observation of films and to obtain a Raman spectrum measurement of buried foreign matter.
↑Observation of multilayer film
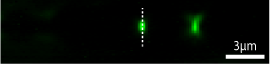
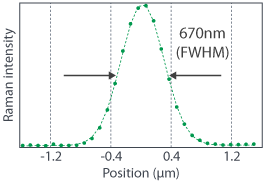
Sample | Fluorescent beads(φ200nm) |
Excitation wavelength | 532nm |
Obj. lens | 100x, 0.90N.A. |
What is Confocal Microscopy?
Confocal Microscopy is an optical imaging technique for increasing optical resolution and contrast by placing a confocal aperture at the confocal plane to eliminate light from outside of the focal point. If the confocal aspect of the microscope is poor, excess Raman signals from above and below the focal plane would appear as background, affecting measurements such as the observation of different layers in multi-layered films or the acquiring of a sample’s weak signals at high sensitivity.
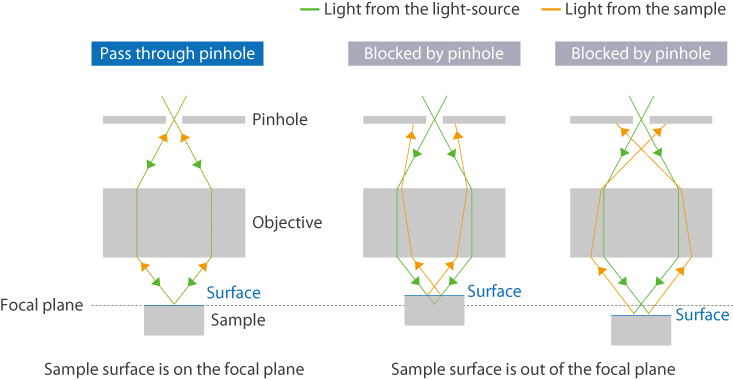
Raman analysis of a 100 nm particle
Our highest spatial resolution and high performance confocal optics enhance our sensitivity to detect tiny particles. The laser beam will be accurately focused on the center of a particle using galvanometer scanner, and a robust sample stage keeps the laser spot always precisely located during long exposure measurements. RAMANtouch/RAMANforce produces a spectrum with a high signal-to-noise ratio that allows particle identification to be done by a library search.
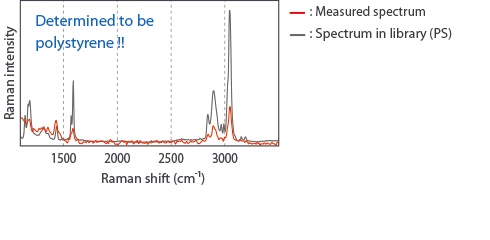
Ultra-fast Raman imaging by line illumination
The dedicated illumination mode developed by Nanophoton has dramatically improved the speed of Raman imaging by re-forming point laser beams into line shapes to cover a larger sample area. This patented line illumination excites Raman scattered light along the line-illuminated area simultaneously whereupon it is divided into 400 spectra by a large number of pixels using a CCD.
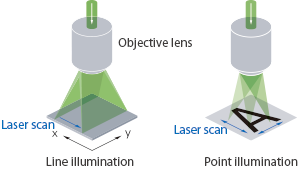
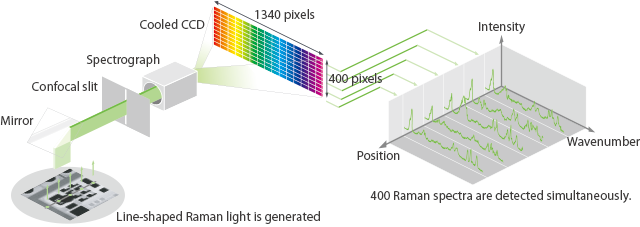
As one of Nanophoton’s specialized technologies RAMANtouch/RAMANforce is equipped with galvanometer scanner for fast and accurate laser beam scanning. Compared with a conventional motorized stage, laser beam scanning performs several hundreds times faster scanning with up to 10 nm positioning accuracy. This also has advantages in operation; e.g. just clicking on the microscopic image will designate the specific area for measurement without any vibrations.
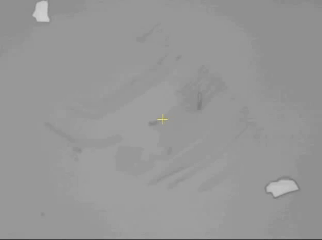
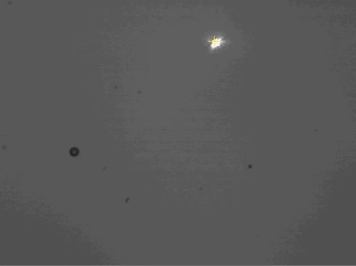
There has been a long-lasting controversy about whether laser scanning technology deforms the laser spot, especially at the edge of the field-of-view. However, using the most updated optics allows focusing of the laser beam perpendicular to the focal plane over the whole area.
Optical system has been redesigned to be 1.93 times more sensitive
All optical components in RAMANtouch/RAMANforce were carefully reviewed and updated using the latest optical materials and coating technologies. In addition, Nanophoton has designed a new spectrograph and adopted the top grade CCD with highest quantum efficiency, thus our updated RAMANtouch/RAMANforce uses new optics with 1.93 times more sensitivity than our previous model.
As the graph shown below, the detection sensitivity of RAMANtouch/RAMANforce is 1.93 times higher than previous model at 100 cm-1 with 532 nm excitation, and 1.86 times higher at 553 cm-1 with 785 nm excitation.
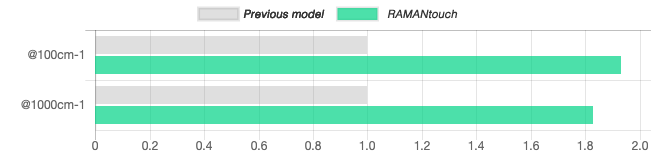
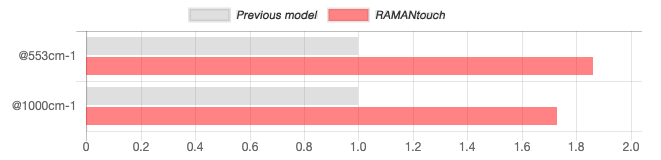